— Extracting quantitative information from microscopy data
We generated the following programmes – all freely available as ImageJ plugins under the “Software” tab in this website:
1. DeadEasy Caspase for the Embryonic CNS and DeadEasy Caspase for the Larval CNS: these two programmes count the number of cells labelled with anti-cleaved-Caspase-3 and with DCp1, which detect apoptotic cells in the entire CNS. (For example of use see: Zhu et al 2008 PLoS Biol and McIlroy et al 2013 Nat Neurosci).
2. DeadEasy Neurons: this programme was developed to count the number of neuronal nuclei in the embryonic CNS labelled with anti-HB9. HB9 nuclei are abundant and quite packed, so this programme should cope well with other nuclear markers of similar characteristics, as long as the image qualities (histogram, background, noise) are comparable.
3. DeadEasy Mitoglia: to count the number of dividing cells with nuclei stained with anti-phospho-Histone-H3 and the number of glial nuclei stained with anti-Repo in the embryonic CNS. Since both of these antibodies result in images with very high signal and very clean, low backround, the programme handles both of these stains well. It is likely that this programme will work well with other nuclear markers that label sparsely distributed nuclei (e.g. BrdU). (For example of use see Learte et al 2008 Glia).
4. DeadEasy Larval Glia: to count the number of glial cells labelled with anti-Repo in the larval CNS. This programme will work well with other nuclear markers that label sparsely distributed nuclei (e.g. pH3 and HB9, see Forero et al 2012). (For example of use see Kato et al 2011 PLoS Biol).
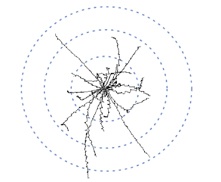
5. DeadEasy Synapse: this was developed to quantify stainings with the nc82 antibody (anti-Brp) in the larval neuro-muscular junction (NMJ), which visualise synaptic active zones, to reveal if a certain genotype compromises synaptogenesis or induces formation of further synapses. Active zones are visualised as small spots with anti-Brp. The programme does not count each individual spot, as we were not able to obtain a satisfactory degree of accuracy and low error. Thus, this programme measures the total volume in 3D occupied by Brp voxels in a stack. (See Sutcliffe et al 2013, PLoS One 8(10): e75902).
6. FlyTracker: we modified an available ImageJ plug-in in order to track larvae crawling in an agar plate, or flies walking along the rim of a petri-dish. The programme measures automatically speed, distance and wobbling. (See McIlroy et al 2013 Nature Neuroscience).
Examples of image types for DeadEasy programmes:
Anti-Cleaved-Caspase-3 labelled embryonic CNS Anti-Repo in a normal embryonic CNS (left) and a larval CNS of a mutant specimen (right) NMJ labelled with anti-Brp (nc82, green), HRP (magenta)
All of our programmes count cells in 3D, i.e. looking at the data throughout the whole stack of sections, not in projections. They produce new stacks, with the same number of images as the original raw data, and in which the cells or flies identified by the programme are shown.
We generated several different DeadEasy programmes to obtain maximum accuracy and minimise the error. The different DeadEasy programmes have been developed specifically to handle the image properties conferred by the different antibodies used. For example, anti-cleaved-Caspase 3 is primarily a cytoplasmic signal, although it can also result in strong pycnotic round cells, but it is in general rather noisy with spotty backgorund. In contrast, anti-Repo is nuclear and very clean, with negligible background. However, counting nuclei is not trivial, and we were not able to develop a satisfyingly good programme that could count all nuclei in the CNS, stained either with nuclear dyes (e.g. DAPI) or with different nuclear antibodies. The problem is that when looking at all nuclei, they lie very tightly packed, close to each other, and the programmes could not identify each nucleus without creating error (i.e. counting too few or too many). To overcome this, and thus not compromise in accuracy, we developed two different programmes that could handle nuclear stains, whilst not counting absolutely all nuclei: one for glial cells, and one for neurons. We also found that if we used the same programme for all developmental stages (embryo, larva and adult) it would also compromise on accuracy and increase the error. Thus we created versions specifically adapted to work optimally at either embryo, larva or adult stages.
All of our programmes have been validated to measure the error (false positives, false negatives and sensitivity) with specimens for which they were developed, and the error for each programme is rather low. All the details of the validation are given in the papers we have published describing the methods. The parameters can be changed to suit other sample types, but in this case, the resulting new versions must be validated.
For full descriptions of the methods see:
Li et al 2020; Forero et al. 2012; Forero and Hidalgo, 2011; Forero et al. 2010; Forero et al. 2010; Forero et al. 2009; McIlroy et al. 2013; Sutcliffe et al. 2012 in our publications tab.
Funded by The Wellcome Trust.
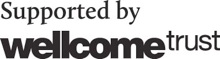